Comments
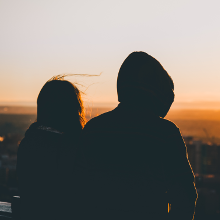
Invited Review Comment #101 Ulf Lorenz @ 2024-03-13 14:03
The paper has definitively been improved. The diversity term is now clear, and with the comments of the authors,
many unclear points could be clarified. Nonetheless, there still are several critical points, on the detail-level as well as on a meta-level.
On meta-level: I understand that it is no scientific contribution in the classical sense, instead the data-generation is in
the center of interest. What makes the data set remarkable? I explain the question.
When Open-AI trains a GPT model and something like GPT-3 comes out, I think it is highly interesting to learn, how that is possible. And therefore the learning data are highly interesting for the scientific world. Or, let us say, someone has written a high-ranked paper and we wonder whether the experiments have really been taken place, maybe because we cannot directly re-build the experiments, then these data are valuable.That are occasions, where a scientic project produces data which are difficult to gather, maybe in thousands of hours.
This is different here, the authors try to sell datasets produced in stockpile. It does not become clear to me what it might be used for. Although some idea is given, this is not tangible enough for my taste, it is by far too hypothetic.I would expect, first to make a point in science or real life, and then to share the data in order to increase credibility and build a platform for further research on it.
On detail level:
- Of course, it is clear what a job-shop problem is and what a flow shop problem is. My question was and is, why you
create a new one for this paper. A flow shop problem is a well known problem in literature. Job shop is
as well. But why do you construct a new one in between? - ll. 82 - 84: "how close can a given non-exact method be expected to come to the optimal solution for a
given problem?" This is the topic of e.g. approximation theory. Indeed there are positive results like a PTAS here:
Jansen, K., Solis-Oba, R., Sviridenko, M. (1999). A Linear Time Approximation Scheme for the Job Shop Scheduling Problem. In: Hochbaum, D.S., Jansen, K., Rolim, J.D.P., Sinclair, A. (eds) Randomization, Approximation, and Combinatorial Optimization. Algorithms and Techniques. RANDOM APPROX 1999 1999. Lecture Notes in Computer Science, vol 1671. Springer, Berlin, Heidelberg. https://doi.org/10.1007/978-3-540-48413-4_19
This belongs to heuristics / algorithms with polynomial time. But even when a PTAS exists, for every heuristic and every natural number k, one can construct an instance which is at least k away from the optimum. That becomes different as soon as you allow more time steps for the algorithm. At the end you can achive an exponential time algorithm which solves to optimality. With a comparable number of time steps, you can of course achive arbitrary
non-exact methods which reach the optimal solution arbitrarily close. - " In other words, what is the expected optimality gap of a method for a specific problem instance." Here, you change your argumentation to instances (away from "problem", which contains infinitively many instances). For single instances, the answer to the previous question ll. 82 - 84 is clear: for each solvable instance, there exists an algorithm which solves it. For any instance you find such an heuristic which makes the job optimal. When you change "instance" to "instance set" it is the same as long the instance set is a finite number of finite instances. As soon as the number of instance sets (all with finite size) is no longer finite, the no free lunch theorem says that there is no heuristic which perfoms gernerally better than others.
"The expected optimality gap of a method for a specific problem instance" may make sense when the probability space is given more precisely. As long as the only restriction against all possible instances is only "the entropy is p", and p from (0,1), it will be as hard to compute this expectation value as without the restriction. If the ground set of all instances is thought to be finite, e.g. a large data base, it is possible, but then extrapolation is highly limited. I.e. the result depends on the concrete examples that you collect for your database, especially it is restricted to instances up to a fixed length. I guess this is what you intend, but I am not sure.
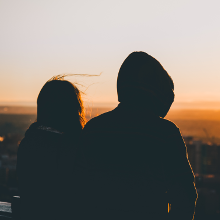
Invited Review Comment #89 Anonymous @ 2024-01-31 14:40
Comment #86 Marco Kemmerling @ 2024-01-21 16:56
Dear Mr. Unger, Dear Reviewers,
we have revised our submissions and give replies to the reviewers' comments below.
-----------------------------------
Response to Review Nr. 1 (Anonymous):
Dear reviewer,
Thank you for your review. We have taken your suggestions into consideration and revised the submission accordingly. Below, we reply to each of your comments point by point.
> Find a source for the universally valid definition of the job shop problem
We have included a source for the definition of the job shop problem.
> line 98: "the number of possible machie types depends on the problem size
The typo has been fixed in the revised submission.
> line 158: repeated until the desired
We have added the missing word.
> line 162 - 164: The sentence appears to be grammatically incorrect and long. Consider splitting it into two sentences for better clarity and readability.
The sentence has been split into parts and reformulated.
> line 163: size of P is much larger
We have added the missing space.
> line 190 be able to deepen the understanding
We have added the missing word.
> Reference the Appendix within the text.
A reference to the appendix has been added at the end of section 2.4.
-----------------------------------
Response to Review Nr. 2 (Ulf Lorenz)
Dear reviewer,
Thank you for the detailed and thoughtful review. You make a number of valid points that have led us to revise parts of our submission to be more clear and understandable. Below, we address each of your criticisms point by point.
> The present draft is not strong enough for a high-level scientific publication. Moreover, the intention of the paper is not clear to me. Is it thought as a demonstration package, how to manage data and program contributions in the ing.grid ecosystem? Or is the intention to present a scientific contribution, and the data and data generator are re- usable part of the paper?
As indicated in the Pre-print, above the title of the submission, our contribution is a dataset descriptor. Its purpose is solely in making research data available to the wider scientific community by presenting a newly generated dataset, motivating the reason for its existence, and describing the generation process. It is not meant to make any scientific contributions beyond the generation of the dataset. Tests of novel scientific hypotheses or analyses of the performance of algorithms on the generated dataset are hence not within the scope of this dataset descriptor, but will be carried out in further publications.
> What I see is an automatically generated collection of quite simple and in a certain sense trivial files. Are these data really what the scientific community needs to get current challenges under control? Will you use these data for your further research?
As indicated in the conclusion, the generated data will indeed be used for further research on the impact of operation and job entropy on the performance of learning-based scheduling approaches. While the structure of the files is fairly simple, the contents of the files fully define a given job shop problem instance and contain comparable information to other common benchmark datasets (see e.g. http://jobshop.jjvh.nl).
> several keywords from various theoretical contexts are mixed into a set of conjectures and claims, but no evidence is given for them.
The dataset described in this dataset descriptor was explicitly designed to test a specific set of claims or hypotheses. These tests are indeed not part of the dataset descriptor, but will be carried in the context of subsequent publications. We have rewritten our submission to make this more apparent.
> Problem definition: the paper starts with the term job shop. In lines 35 to 47 it is defined. I do not understand why the authors go via |O_j| = |O| / |J|. As is said, the point is that all jobs have the same amount of operations and there are exactly as many machines as each job has operations. What is the difference to the Flowshop problem? There is vast literature on flow shops, which examines which subproblems stay NP-hard and which are solvable in polynomial time, the oldest go back to the 70s [3]. If you have something in mind which makes your problem different from Flowshop, why do you invent a new similar special case?
The flow shop problem fundamentally models assembly line production, whereas the job shop problem models job shop production. This means that in a flow shop problem, the operations of each job are processed on the same machines in the same order. In a job shop problem, an individual order of machines can be specified for each job. Our dataset is concerned with the latter case. The decision of making all jobs have a size equal to the number of machines is simply a common assumption (see e.g. Taillard (1993) “Benchmarks for basic scheduling problems” and Samsonov et al. (2022) “Reinforcement Learning in Manufacturing Control: Baselines, challenges and ways forward”). In the absence of this assumption, additional degrees of freedom emerge, which will have to be accounted for in both the generation of the data and also in any experiments that will be carried out using the datasets. Since the length of jobs is not a property of interest in our datasets, we believe it is prudent to keep it constant, so that its (potential) effects do not interfere with the (potential) effects of our property of interest (entropy).
> CP 1: It is claimed that the entropy as defined in sections 2.1 and 2.3 is a good formalization for diversity, itself an intuitive term that is described in section 2.1, lines 49 - 56. The text contains several attempts to explain what the intuitive meaning of „diversity“ should mean and what the connection to entropy is, but I do not understand this point, nonetheless. To the best of my knowledge, maximum entropy just means that all symbols (jobs / operations in this case) are complete random, i.e. are equally distributed. (Cf. [4]). Thus, if one wants to generate instances with “maximum entropy“, just sample randomly, or equally distributed respectively.
Sampling randomly would not necessarily generate instances with maximum entropy (in terms of the operation and job entropy we have introduced in our submission), as the same sample could be drawn multiple times by chance, even when sampling from a maximum entropy distribution. The introduced entropy measures describe the characteristics of (sets of) instances, not probability distributions from which instances may be sampled. The difference here is that the samples have already been drawn and we measure frequencies within those samples. Essentially, we view a job shop problem instance as a discrete probability distribution, so that each unique operation corresponds to an event, and the frequency of this operation in the problem instances corresponds to the probability of the event. We can then measure the Shannon entropy of the probability distribution derived from the problem instance. We have clarified this difference as well as the meaning of diversity in the revised submission.
Additionally, the maximum entropy case is a trivial one. The goal of our generation procedure is to generate many different instances which inhabit the complete continuum between minimum and maximum entropy. The more unique operations are present in a given instance (the higher the diversity of operations is), the higher the operation entropy will be, which simply follows from the definition of the Shannon entropy. Further, the reference point (base of the logarithm) for the calculated entropy is always the total number of operations. This means that you could have two unique operations within a total of 100 operations and these two unique operations might be equally distributed such that each occurs 50 times. Such an instance could be sampled from a maximum entropy probability distribution, but the instance itself would still be a low operation entropy problem instance. The maximum operation entropy would be achieved with 100 unique operations, each occurring exactly once.
> A question may remain whether it may make any sense to construct instance sets with low(er) entropy. However, when you build instance sets with a pre-given entropy, you do nothing else than constructing instance sets with arbitrary non-uniform distributions. The probability that a sample procedure produces non-desired samples will certainly converge to zero fast, with increasing number and sizes of the sample points, i.e. generated instances. But why should that coincide with the desired “diversity”? I cannot see this, although I am aware that you are neither the first who construct entropy based test data [6, 7], nor you are the first who claim a connection between the word diversity and your given formulas [9]. In [9], the term diversity is covered and discussed in a quite sophisticated and systematic way. I do not understand, why you think that the effect above, my little example with the english language, should not hold true for your generator as well. Why do you think your generator generates anything meaningful in spite of this sobering observation?
Although the abstract nature of job shop instances sets them apart from natural language sentences, the criticism is not completely without merit. It cannot be ruled out that the instances generated by us differ in some important way from instances as encountered in real production scenarios. However, this is not a criticism that is specific to our instances, but it concerns artificially generated instances in general. For instance, the well-known Taillard benchmark contains instances created by random sampling, and the meaningfulness of the results is hence similarly questionable.
For our purposes, this is not especially problematic, although the job shop scheduling community should generally keep in mind that artificially generated datasets may exhibit different properties from those encountered in reality. We aim to provide datasets that can be used to investigate the effect of the proposed entropy measures. In a real production scenario, the product mix determines the diversity of jobs and operations and different production scenarios will hence lead to different job and operation entropy characteristics. Results from artificially generated datasets with different entropy characteristics could then predict how different scheduling approaches would perform in real production scenarios with similar entropy characteristics. Whether the results transfer from the artificial to the real would naturally have to be confirmed experimentally.
Although caution is advised when working with artificial data, it nevertheless holds great value for job shop scheduling research for two reasons: (1) the lack of publicly available real production scheduling instances and (2) the ability to vary instance properties in a controlled manner.
> CP 2: It is claimed that the entropy may give a hint on easy and hard instances. Also for this conjecture, the paper contains no evidence, except the trivial observation that a minimum-entropy instance is trivial. This may indeed be an exceptional or even the only example where this fits. The term difficulty is not well defined in the paper, but if we follow what other authors have been interested in, [2] may help.
The terms “easy” and “hard” are indeed not well defined in the submission and may lead to confusion due to conflation with existing terms such as “NP-hardness”. We have clarified what we mean by these terms in the revised version of the submission. The intended meaning is concerned with how close non-exact approaches are expected to come to the optimum solution, i.e. how large the optimality gap is expected to be. If the optimality gap is larger, the instance may then be considered to be more difficult.
While it is true that the paper contains no evidence for the conjecture, this is by design. Since the paper is a data descriptor, it only provides the basis on which experiments can be performed. The generation of the basis for the experiments must necessarily precede the experiments and the derivation of results themselves. If the results were already known, there would be no reason to generate a dataset.
In case it is helpful to know for the review process, we have already performed experiments that show increasing optimality gaps with increasing operation entropy in solutions generated by scheduling agents. We are hence confident that the entropy measures introduced here show some usefulness in the characterisation of job shop instances. Nevertheless, as this is merely a dataset descriptor, we feel that the inclusion of these results in the submission would be inappropriate. However, publication of these results in the form of a regular scientific paper is planned.
> CP 3: You claim that all this is motivated from your research on machine learning. I can believe this or not, there are no hints that this is correct. If you could show me experiments which indicate that the learning rate within a machine learning experiment brings better or faster results if you decrease the entropy in a certain way, compared to random sampling, I would says, “ok this is a result”. But it is not part of the paper.
See above. If we included experiments already, the paper would not be a dataset descriptor anymore, but would simply be a regular scientific paper.
> CP 4: I do not understand what the neural network in section 3 is good for. If there is a pre-given dataset behind, it should not be difficult to compute the entropy and generate new instances. If there is no such pre-given database, what does it learn? Moreover, when the entropy value is pre-given, there are many possibilities to construct probability distribution which all have the desired entropy value. To which one do you go? Any arbitrary one?
A pre-given dataset does not exist at this point. The purpose of the neural network is to generate a probability distribution from which problem instances can then be sampled. This is especially relevant for the inter-instance datasets, where we aim to generate different problem instances which originate from a common distribution. For a given dataset / problem instance, the choice of distribution is indeed arbitrary as long as the desired entropy value is achieved. Of course, we generate many such datasets / instances, each with different underlying distributions.
Note that this could certainly be achieved by more elegant and efficient means. However, the code is meant to be executed exactly once, to generate the data. Elegance and efficiency were hence not a priority, and our approach was simply chosen due to its ease of modelling and the convenience associated with modern automatic differentiation frameworks. We have clarified this in the submission.
We hope that our revisions are to your satisfaction and again thank you for the detailed review.
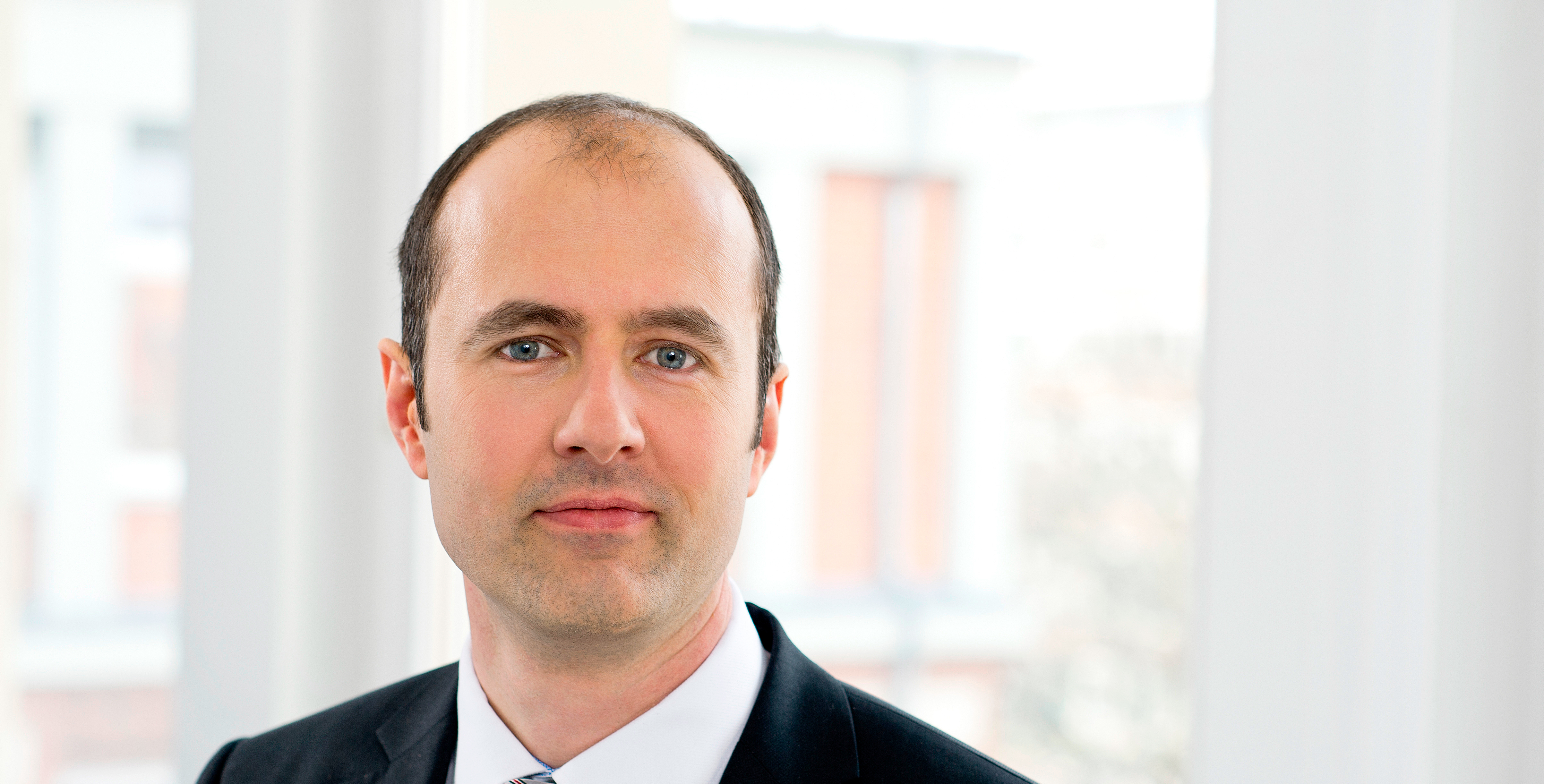
Comment #78 Jörg F. Unger @ 2023-12-20 15:12
Dear Mr. Kemmerling,
the reviews of your paper "Job and Operation Entropy in Job Shop Scheduling: A Dataset" are now publicly available. In the current form, the paper/dataset can not be published in ING.GRID. Please reply to the comments from the reviewers and revise the manuscript.
Best regards,
Jörg F. Unger (topical editor)
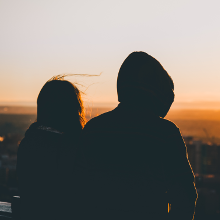
Invited Review Comment #75 Ulf Lorenz @ 2023-12-12 16:14
Review for Ing.grid
Topic: test data generation, scientific contribution
Paper:
Marco Kemmerling , Maciej Combrzynski-Nogala, Aymen Gannouni, Anas Abdelrazeq , Robert Heinrich Schmitt: Job and Operation Entropy in Job Shop Scheduling: A Dataset
Reviewer:
Ulf Lorenz
Total judgement:
The present draft is not strong enough for a high-level scientific publication. Moreover, the intention of the paper is not clear to me. Is it thought as a demonstration package, how to manage data and program contributions in the ing.grid ecosystem? Or is the intention to present a scientific contribution, and the data and data generator are re- usable part of the paper?
Reasoning for judgement:
The paper consists of two parts. One regards a set of benchmark instances of the job shop problem. The key contribution is to generate a test dataset and to share the instances as well as the generator with the scientific community. The other part consists of a supporting scientific text that is intended to motivate and describe the new benchmark set. The idea here is to establish Shannon entropy as a measure for diversity of benchmark instances, and for difficulty inside computational complexity theory.
I start with the second part, the dataset contribution. When we follow the dataset link tohttps://zenodo.org/records/8288814 or the git-repository link https://git.rwth- aachen.de/jobshop/entropy, we see a perfectly organized presentation for both. Everything is nicely arranged and well commented. If my interest were to re-use this contribution, I will certainly be success- and thankful. However, the presentation itself seems to be the most expensive part of the entire package. The python-script is proper and well done, but not really demanding. A similar impression arises when inspecting the data. What I see is an automatically generated collection of quite simple and in a certain sense trivial files. Are these data really what the scientific community needs to get current challenges under control? Will you use these data for your further research?
The paper itself starts with the job shop problem and a complexity classification.
Preliminary remark: Computational complexity theory is the most influencing theory within the last decades and has led to enormous progress in algorithmics and in understanding the difficulty of problems. The most impressive insight from my point of view is that problems can be partitioned into classes, and inside any class, all problems can easily be converted into each other. And, by the way, such a conversion, called a reduction, does not conserve entropy, which leads to a second theoretical concept. The Shannon entropy stems from information theory which can be seen complementary to computational complexity theory. Computational complexity theory typically is more rigorous. E.g., if one wants to compress a textile, one can count how often which symbol arises and, assign bit pattern to the symbols in accordance to the entropy. The result is e.g. a Huffman-encoding. However, this should not be confused with a smallest encoding at all. To decide what a smallest possible encoding is, is undecidable, cf. Kolmogorov complexity.
To stay in this line with texts, it is also clear that a generator for texts, which is based on entropy only, will nearly never produce meaningful english text, even if the symbol generator follows the correct probabilities of symbols. If only the entropy value itself is given, the generator cannot even produce a symbol chain with the correct probabilities, because there are many probability distributions with the same entropy value.
To the paper draft: As a consequence, I have several critical remarks. Some have their origin from the fact that I simply do not understand the authors’ intentions, this might easily be repaired with a short remark. Others seem to have deeper impact, I fear. My main concerns with this paper are
- several keywords from various theoretical contexts are mixed into a set of conjectures and claims, but no evidence is given for them. The major keywords are entropy, diversity, complexity, difficulty, neural networks.
- an appropriate literature overview should be part of a scientific paper, firstly to show that the authors know what they talk about, and secondly to give an insight how the own contribution is to be classified.
Problem definition: the paper starts with the term job shop. In lines 35 to 47 it is defined. I do not understand why the authors go via |O_j| = |O| / |J|. As is said, the point is that all jobs have the same amount of operations and there are exactly as many machines as each job has operations. What is the difference to the Flowshop problem? There is vast literature on flow shops, which examines which subproblems stay NP-hard and which are solvable in polynomial time, the oldest go back to the 70s [3]. If you have something in mind which makes your problem different from Flowshop, why do you invent a new similar special case?
More critical points (CP 1 ... CP 4) are as follows.
CP 1: It is claimed that the entropy as defined in sections 2.1 and 2.3 is a good formalization for diversity, itself an intuitive term that is described in section 2.1, lines 49 - 56. The text contains several attempts to explain what the intuitive meaning of „diversity“ should mean and what the connection to entropy is, but I do not understand this point, nonetheless. To the best of my knowledge, maximum entropy just means that all symbols (jobs / operations in this case) are complete random, i.e. are equally distributed. (Cf. [4]). Thus, if one wants to generate instances with “maximum entropy“, just sample randomly, or equally distributed respectively.
A question may remain whether it may make any sense to construct instance sets with low(er) entropy. However, when you build instance sets with a pre-given entropy, you do nothing else than constructing instance sets with arbitrary non-uniform distributions. The probability that a sample procedure produces non-desired samples will certainly converge to zero fast, with increasing number and sizes of the sample points, i.e. generated instances. But why should that coincide with the desired “diversity”? I cannot see this, although I am aware that you are neither the first who construct entropy based test data [6, 7], nor you are the first who claim a connection between the word diversity and your given formulas [9]. In [9], the term diversity is covered and discussed in a quite sophisticated and systematic way.
I do not understand, why you think that the effect above, my little example with the english language, should not hold true for your generator as well. Why do you think your generator generates anything meaningful in spite of this sobering observation?
CP 2: It is claimed that the entropy may give a hint on easy and hard instances. Also for this conjecture, the paper contains no evidence, except the trivial observation that a minimum-entropy instance is trivial. This may indeed be an exceptional or even the only example where this fits. The term difficulty is not well defined in the paper, but if we follow what other authors have been interested in, [2] may help.
With this in mind, there is not much hope for the conjecture, and I claim that it is wrong. The No-Free-Lunch-Theorem [3] states that there exists no dominating algorithm. A major consequence certainly is that the term „difficulty“ cannot be covered without its dependency of algorithms - which certainly also is the reason why the authors in the paper [8] (which you cite as well), followed another approach.
In relation to computational complexity, the situation is as follows. As soon as you have decided for a probability distribution with given entropy, all probabilities are fixed. These probabilities do not exclude instances (as long as p_i > 0) and will therefore not influence the worst-case complexity of the problem, assuming infinitely large instance sets are generated. There may be a chance to influence the average-case complexity of the job-shop problem, but I see no evidence, not even hints, for this. However, there are papers, dealing with similar questions [1].
Last but not least, there is the following simple thought experiment. When you define a Flowshop (sub-) problem and you allow m machines, you decrease the entropy when you forbid some of the m machines. You can do that until you have three machines, and although the entropy decreases, the complexity class stays NP-hard. When you go down to 2 machines, the problem moves from NP to P. However, you can increase the number of machines after that again, and build a subset with machines that can do only trivial things, and the complexity class stays P. Entropy can just not cover how complicate the job structure is.
CP 3: You claim that all this is motivated from your research on machine learning. I can believe this or not, there are no hints that this is correct. If you could show me experiments which indicate that the learning rate within a machine learning experiment brings better or faster results if you decrease the entropy in a certain way, compared to random sampling, I would says, “ok this is a result”. But it is not part of the paper.
After all, the Shannon entropy is a flat measure for complexity, similar as the pure size of a search space, sometimes called the combinatorial explosion. Also the latter has not much to do with problem complexity. There are problems with exponential large search spaces (measured in bits of the input length) which can be solved in polynomial time and there are others which are NP-hard or harder. And there are (infinitely-large) subsets of problem instances generated with low entropy that are hard and there are some with high entropy, but nonetheless easily solvable.
CP 4: I do not understand what the neural network in section 3 is good for. If there is a pre-given dataset behind, it should not be difficult to compute the entropy and generate new instances. If there is no such pre-given database, what does it learn? Moreover, when the entropy value is pre-given, there are many possibilities to construct probability distribution which all have the desired entropy value. To which one do you go? Any arbitrary one?
[1] Scharbrodt, Mark and Schickinger, Thomas and Steger, Angelika. A New Average Case Analysis for Completion Time Scheduling, Association for Computing Machinery, Volume 53 Issue 1, 2006
[2] Kate Smith-Miles, Leo Lopes. Measuring instance difficulty for combinatorial optimization problems, Computers & Operations Research, Volume 39, Issue 5, pp. 875-889, 2012
[3] Garey, M. R., Johnson, D. S., & Sethi, R. (1976). The Complexity of Flowshop and Jobshop Scheduling. Mathematics of Operations Research, 1(2), 117–129. http://www.jstor.org/stable/3689278
[4] https://en.wikipedia.org/wiki/Entropy, for overview, especially information theory) [5] https://cognitivemedium.com/magic_paper/assets/Hornik.pdf
[6] a simple google-inquiry: https://www.google.de/search?q=using+entropy+based+sampling+to+generate+test+data&sca_ esv=588668658&ei=i4pxZZDzG5qF9u8PyNahsAM&ved=0ahUKEwjQ5JmC_PyCAxWagv0HHU hrCDYQ4dUDCA8&uact=5&oq=using+entropy+based+sampling+to+generate+test+data&gs_lp =Egxnd3Mtd2l6LXNlcnAiMnVzaW5nIGVudHJvcHkgYmFzZWQgc2FtcGxpbmcgdG8gZ2VuZXJh dGUgdGVzdCBkYXRhMgoQIRigARjDBBgKMgoQIRigARjDBBgKMgoQIRigARjDBBgKSJcnUJ4 PWOMgcAF4AZABAJgBeaABqwmqAQQxMS4zuAEDyAEA- AEBwgIKEAAYRxjWBBiwA8ICCBAhGKABGMMEwgIIEAAYgAQYogTiAwQYACBBiAYBkAYI&scl ient=gws-wiz-serp
[7] https://arxiv.org/pdf/1912.08920.pdf
[8] C. Waubert de Puiseau, H. Tercan, and T. Meisen, “Curriculum Learning In Job Shop Scheduling Using Reinforcement Learning,” in Proceedings of the Conference on Production Systems and Logistics: CPSL 2023, vol. 4, 2023, pp. 34–43.
[9] Jost, L. (2006), Entropy and diversity. Oikos, 113: 363- 375. https://doi.org/10.1111/j.2006.0030-1299.14714.x
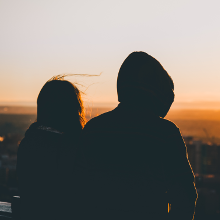
Invited Review Comment #73 Anonymous @ 2023-11-30 17:05
Proposed revisions:
Chapter 2
- Find a source for the universally valid definition of the job shop problem
Chapter 2.4
- line 98: "the number of possible machie types depends on the problem size
Chapter 3.2
- line 158: repeated until the desired
Chapter 3.3
- line 162 - 164: The sentence appears to be grammatically incorrect and long. Consider splitting it into two sentences for better clarity and readability.
- line 163: size of P is much larger
Chapter 4
- line 190 be able to deepen the understanding
Reference the Appendix within the text.