Comments
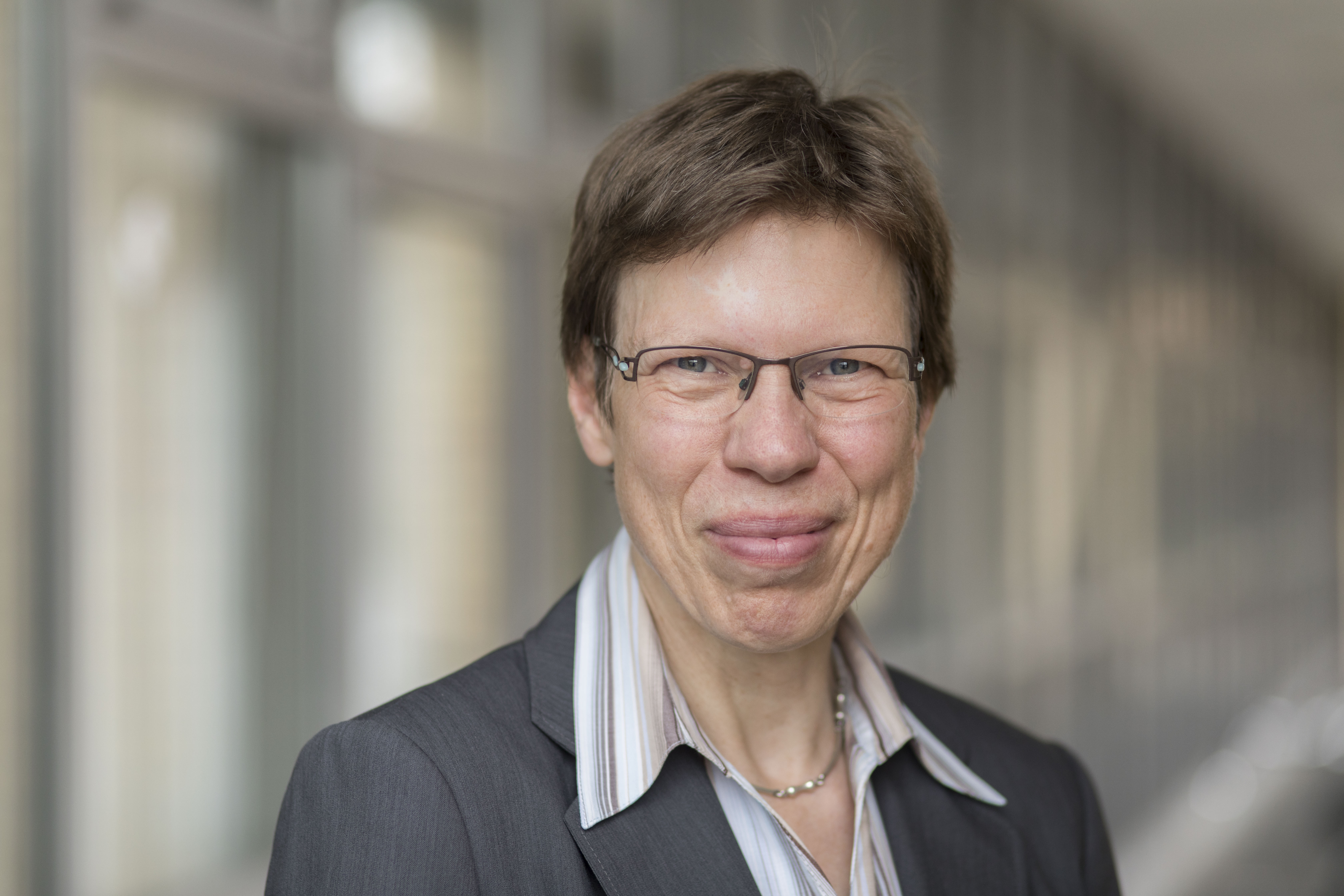
Comment #107 Irina Sens @ 2024-04-18 10:42
Ready to publish. Thanky to the reveiwes and the authors.
Invited Review Comment #100 Björn Schembera @ 2024-03-11 16:02
The manuscript is now in a good state, as it is now clearer to the reader why ethics are important for data literacy.
Some minor comments that should be adressed are:
- Usually, abstracts don't need references.
- Sometimes, "et. al." is without th full stops
- The reference boxes are sometimes not sperated by a space from the text
- Line 31: Comma is wrong
- Line 45: Card sorting might not be known to the reader. Please add sentence on this method.
Moreover, one could mention in the outlook that quite recently, the ethics working group of the ELSA-section in the NFDI has been instantiated, see here:
https://www.nfdi.de/tf-ethik-workshop-februar-2024/
In this working group, such topics could be further worked out.
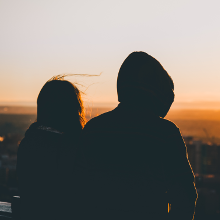
Invited Review Comment #94 Anonymous @ 2024-02-14 09:10
The paper deals with questions of "research ethics" (here simply called "ethics"). On an empirical basis (focus group), it promotes a broader understanding of research ethics as part of data literacy/data science, primarily for mechanical engineering.
An existing deficit in the literature and the chosen methodological approach are plausibly described, so that the result obtained can be classified. The description of the need for ethics as a need for "critical thinking" (line 19) or an "invitation to reflect" (lines 31/32) is good and sensible.
The article highlights a challenge, it does not offer any solutions. Nevertheless, it is informative insofar as it describes a need - on a small empirical basis, but methodologically with sufficient caution. The following points should be noted before publication:
- The term "ethical problems" should be used throughout the text, rather than "ethical dilemma". A dilemma is the choice between two logically contradictory alternatives. Simple 'how to' questions are not dilemmas.
- There is a gap in the manuscript in lines 60-62. - Please complete!
- Capitalization should be used in line 229 (title of the paper).
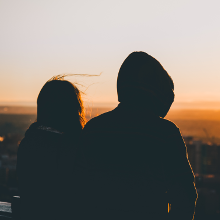
Comment #74 Samira Khodaei @ 2023-12-04 09:34
Dear Topical Editor, Thank you for the chance to rework the submission.
Dear Reviewers, thank you for your valuable feedback! The authors are convinced that your feedback significantly improved the quality of the publication. The comments resulted in many changes to the manuscript. A new version of the submission has been uploaded.
The changes include:
In the following, a reply for each of the major and minor points of the reviewers:
-----
Reviewer 1 Point 1 - Adding Table for Question in the Appendix: Thank you for the suggestion. A table is now added in the appendix.
Reviewer 1 Point 2 - Explanation of the six categories: Further explanation towards the used is added in line 109
Reviewer 1 Point 3 – “In the paper it is never - and this is a weakness - really defined and rolled out what is meant by ethics.” : The definition of ethics is further expanded starting from line 9f.
Reviewer 1 Point 4: -- “[…] why a distinction is made at all between "process-centered" and "human-centered" categories” : Further explaining the categories. Process in distinction to human centered. See Line 123
------
Reviewer 2 Point 1 - Unclear objective: As the objective relates to the question on what are the ethical questions that data scientist face in their daily work. Through this study educational programs on data literacy gain further practice oriented themes to discuss. It is specified in line 31.
Reviewer 2 Point 2 - Ethics in data literacy should be defined in correspondence with research ethics : Added further sources and explanation from data literacy starting in line 9 and line 23 and line 28.
Reviewer 2 Point 3 – “The methodological goal (and purpose) of using the focus group was not clear to me.” : Methodical goal is further explained both in the figure 1 as well as line 88.
Reviewer 2 Point 4 - Suggestion of different approach in method: As clarified in line 31, the objective of this paper is to bridge between theories on data ethics and how much of these theoretical ethics frameworks are reflected in practice among researchers working with data.
Thus, it is mainly about collecting experiences on ethical dilemmas that occur when working with data (existing frameworks). While there are numerous alternative methods that could potentially also reach this goal (e.g. questionnaires, experiments, etc.), we decided to apply the focus group study in order to enable exchange among the
data scientist and people who work with data, finding out whether the ethical standpoints formulated in literature hold true.
Categories that reflect the FAIR principles, point towards the relevance of those concepts. However, there are also newer insights e.g. many participants discussed to which authority one should turn with an ethical dilemma.
The selected focus group study as a method must be open in order to prevent priming the participants and thus results of the study.
These points are discussed in line 81f and 89f.
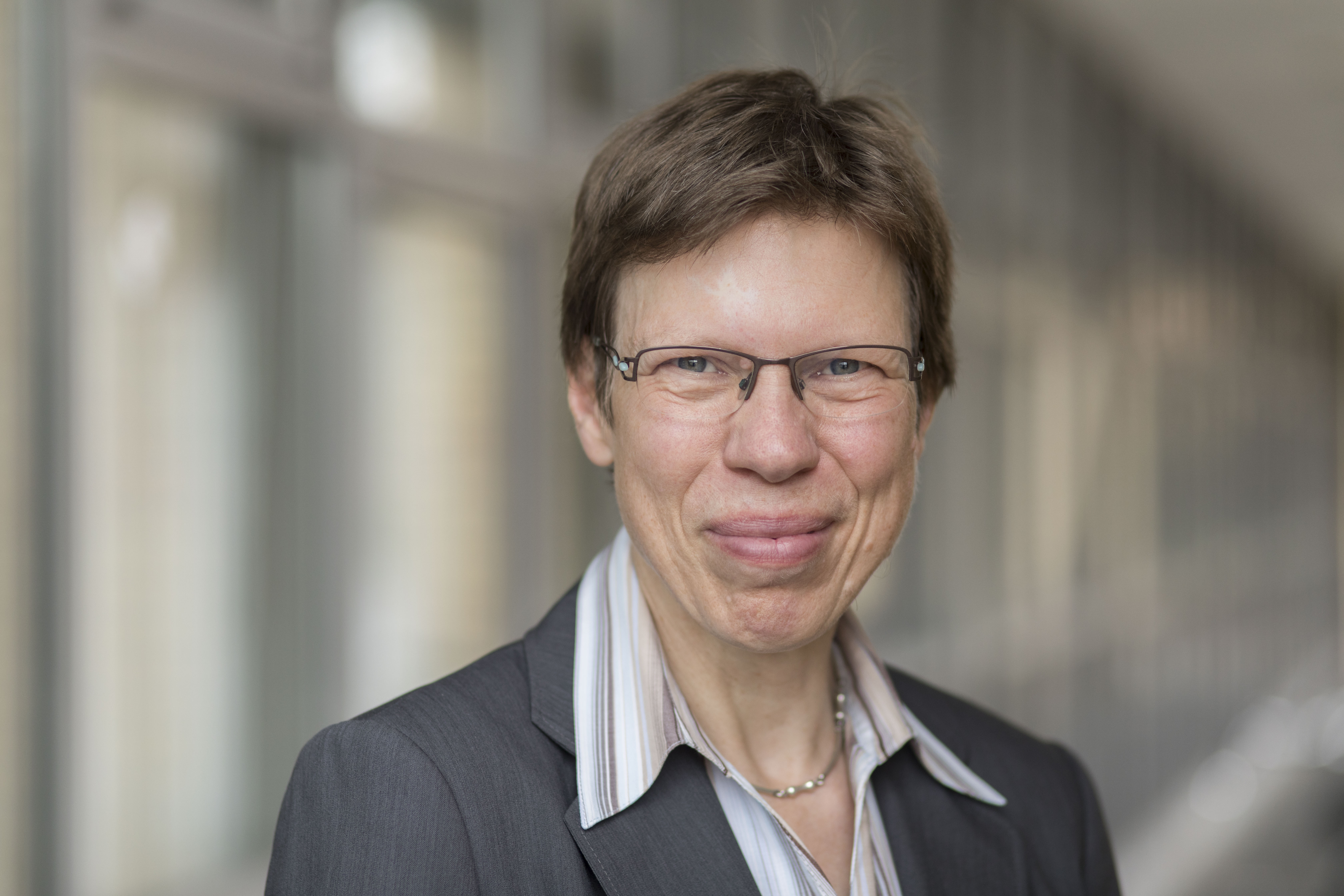
Comment #62 Irina Sens @ 2023-10-18 16:28
As the responsible topical editor, I would like to thank both reviewers for their detailed and constructive feedback. After consideration of the comments, I advise the authors to revise both the descriptor and the repository according to the suggestions provided in the reviews. After completion, the new version of the descriptor including the revisions may be submitted by the authors for further consideration.
Invited Review Comment #42 Björn Schembera @ 2023-08-07 12:37
The paper problematizes that ethics does not appear in any or only a few curricula in the field of data literacy. However, this is important in order to enable critical reflection on data.
The paper makes an important contribution by presenting the results of a workshop that served to collect and categorize ethical issues related to data science. It is overall important that the ethical questions get more attention, especially in the data engineering / literacy domain.
The presentation of the state of the art in section 2 of the paper is convincing, as is the presentation of the methodology in 3.
Areas for improvement of the paper would be as follows:
- The study collected 20 ethical questions. These are mentioned only sporadically in the body text. It would be interesting to see all questions in a table with their classification, or in an appendix (there should be space for this).
- The 6 categories "fall from the sky": Where does it come from? This should be explained.
- In the paper it is never - and this is a weakness - really defined and rolled out what is meant by ethics. However, this would be important for categorizing the questions.
- Following on from this, there is also the question of why a distinction is made at all between "process-centered" and "human centered" categories. Actually, ethical judgments or actions in general can only be carried out by humans (if necessary, of course, mediated by a machine or technology in general), but ultimately it is the human being who decides how to act (which can then be ethically evaluated).
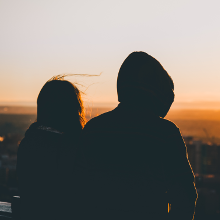
Invited Review Comment #40 Anonymous @ 2023-07-27 13:44
(1) In general, the article (apart from the problems mentioned under (2), (3) and (4)) is not clear enough regarding the objective: is it only about data literacy for mechanical engineering or about data literacy in general?
(2) In my opinion, the keyword "ethics", which is used sweepingly in this article, needs to be specified, otherwise the topic will be missed. In the context of data literacy (for mechanical engineering), it is not about ethics in general, but can only be about "research ethics". This in turn is closely related to issues of "good scientific practice" (Gute wissenschaftliche Praxis, vgl. DFG) and quality assurance in data management and data science. Avoiding biases in data sets, for example, is primarily a question of quality assurance, but not of research ethics. The degree of transparency of data should in turn be set out in a policy of the data-holding institution, etc. (research ethics would require: know the policy, follow the policy or formulate a policy). In this respect, the ethics definition from footnote 8 is simply wrong or unusable. The net publication cited there is not a scientific (and not a suitable) as well as poor quality source on the topic. A topic like corporate power, on the other hand, is a political, not an ethical problem - whereas "digital sovereignty" has meanwhile become a quality criterion of data services and software solutions. E.g., an open source preference may apply to data infrastructures (or an obligation to be GAIA-X compliant). But then it is a defined, "hard" requirement and not ethics.
(3) The methodological goal (and purpose) of using the focus group was not clear to me. Apparently, practitioners were asked about "ethical" concerns/problems at the meeting. As it seems, this was done, however, without defining "ethical" beforehand (or even narrowing the topic to research ethics). The collection of problems that came about in this way thus, unsurprisingly, merely reflects the - apparently amateurish and highly disparate - "general" understanding of ethics of the people involved. What has been collected in this way? At any rate, not any components of a possible research ethics curriculum. Also not really a "need". But actually only a (mis)interpretation of the word (for more an already trained group with an understandig of already established ethics requirements would have been necessary).
(4) The summary of the results of the experiment is correspondingly vague in the text. I do not think that the "collected ethical questions" can point the way to a reasonable "ethics"-extension of data literacy. The method does not fit the goal. And, more importantly, there is not really a need for "categorizing" bottom up. You need to know practice very well to postulate (or to teach) research ethics criteria, but your cannot derive research ethics criteria from empiricism or by collecting opinions. A better approach would be to systematically evaluate the already existing research ethical codes of other data-driven disciplinary cultures and apply them to the research reality of Mechanical Engineering (by experts). Central should also be the (research) ethics specifications of the major engineering associations (in Germany: VDI). Initial AI research ethics guidelines are also already available.